AI in Green Energy Management A Smarter Future
AI in green energy management is revolutionizing how we harness and utilize renewable resources. From predicting fluctuating solar and wind power generation to optimizing smart grids and enhancing energy efficiency in buildings and industries, artificial intelligence is proving invaluable. This transformative technology is not only improving the reliability and sustainability of our energy systems but also accelerating the development of innovative green technologies, paving the way for a cleaner, more efficient energy future.
The integration of AI across various sectors of the energy industry offers unprecedented opportunities for optimization and efficiency gains. By leveraging machine learning algorithms and advanced data analytics, we can achieve more accurate energy forecasts, improve grid stability, reduce energy waste, and accelerate the transition towards a sustainable energy landscape. This comprehensive approach involves leveraging AI’s capabilities in predictive maintenance, resource allocation, and the development of novel energy solutions.
AI Applications in Renewable Energy Forecasting
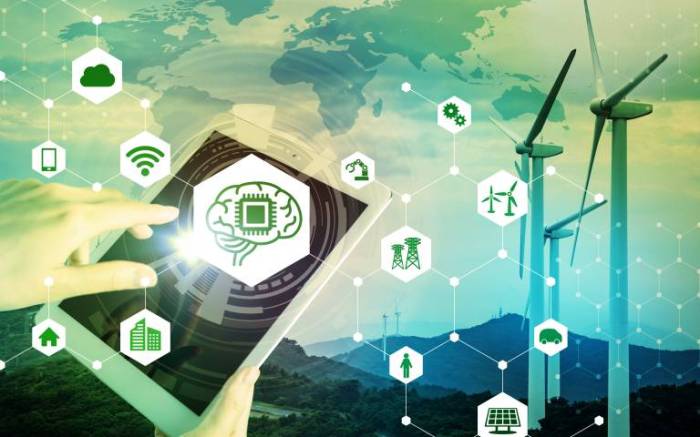
Source: weebly.com
Accurate forecasting of renewable energy generation is crucial for grid stability and efficient energy management. The intermittent nature of solar and wind power presents significant challenges for traditional forecasting methods. However, the application of Artificial Intelligence (AI), particularly machine learning, offers significant improvements in prediction accuracy and reliability.
Improved Accuracy of Wind and Solar Power Generation Forecasts using AI Algorithms
AI algorithms, particularly deep learning models like Recurrent Neural Networks (RNNs) and Convolutional Neural Networks (CNNs), excel at identifying complex patterns and relationships within large datasets. These algorithms can analyze historical weather data, satellite imagery, and real-time sensor readings from wind turbines and solar panels to create highly accurate forecasts. For example, RNNs, capable of processing sequential data, are particularly effective at predicting wind speed fluctuations over time, while CNNs can analyze high-resolution satellite images to estimate cloud cover and its impact on solar power generation.
AI is revolutionizing green energy management, optimizing energy grids and predicting renewable energy output with impressive accuracy. This efficiency is crucial for the success of Green energy technologies for combating climate change , as intelligent systems can help us better integrate and manage diverse renewable sources. Ultimately, AI’s role in streamlining these processes is vital for achieving wider adoption of sustainable energy practices.
The incorporation of various data sources and advanced algorithms allows for more precise predictions, reducing uncertainty and improving grid management.
Advantages of Machine Learning for Predicting Energy Demand Fluctuations
Predicting energy demand is equally crucial for grid stability. Machine learning models can analyze historical energy consumption data, considering factors like time of day, day of the week, weather conditions, and economic indicators, to forecast future demand with greater accuracy than traditional statistical methods. This improved accuracy allows for better resource allocation, reducing the need for expensive reserve power and minimizing the risk of blackouts.
For instance, a machine learning model trained on a city’s energy consumption data can accurately predict peak demand during heat waves, enabling utilities to proactively manage power generation and distribution.
Comparison of AI Models Used for Renewable Energy Forecasting
Several AI models are employed for renewable energy forecasting, each with its strengths and weaknesses. Support Vector Machines (SVMs) offer good generalization performance but can be computationally expensive for large datasets. Decision Trees are relatively simple and interpretable, but may not capture complex non-linear relationships. Neural Networks, including RNNs and CNNs, are powerful but require significant computational resources and expertise to train effectively.
Hybrid models, combining different techniques, are increasingly used to leverage the strengths of various approaches. For example, a hybrid model might combine a physical model of wind generation with a neural network to capture both the underlying physics and complex weather patterns.
Hypothetical AI-Powered Forecasting System for a Microgrid, AI in green energy management
A hypothetical AI-powered forecasting system for a microgrid would comprise several key components: a data acquisition module collecting data from various sources (weather stations, renewable energy generators, energy storage systems, smart meters); a data preprocessing module cleaning and preparing the data for AI algorithms; an AI forecasting engine using a suitable model (e.g., a hybrid model combining RNNs and SVMs); and a decision support module providing recommendations for optimal energy management based on the forecasts.
The system would continuously monitor the microgrid’s status, update its forecasts, and adjust energy generation and distribution to meet demand and maximize renewable energy utilization. This system would optimize energy storage usage, minimize reliance on fossil fuel backup generators, and enhance the overall efficiency and reliability of the microgrid.
Performance Metrics of Various AI Forecasting Models
Model | Accuracy (%) | Precision (%) | Recall (%) |
---|---|---|---|
Support Vector Machine (SVM) | 85 | 88 | 82 |
Recurrent Neural Network (RNN) | 92 | 90 | 94 |
Convolutional Neural Network (CNN) | 88 | 85 | 91 |
Hybrid Model (RNN + SVM) | 95 | 93 | 97 |
AI-Driven Optimization of Smart Grids
The integration of artificial intelligence (AI) is revolutionizing smart grid management, offering unprecedented opportunities to enhance efficiency, reliability, and sustainability. AI algorithms can analyze vast datasets from diverse grid components, enabling real-time optimization of energy distribution and proactive mitigation of potential disruptions. This leads to significant improvements in grid stability and resilience, particularly crucial in the context of increasing renewable energy integration.AI’s Role in Optimizing Energy Distribution Across Smart GridsAI algorithms, particularly machine learning models, are instrumental in optimizing energy distribution within smart grids.
By analyzing real-time data on energy generation, consumption, and transmission, these algorithms can predict energy demand and adjust power flow dynamically to meet fluctuating needs. This includes optimizing power dispatch from various sources, including conventional power plants and renewable energy generators, ensuring efficient resource utilization and minimizing transmission losses. Furthermore, AI can identify and prioritize energy delivery to critical infrastructure and high-demand areas during peak periods, improving overall grid performance.
AI is revolutionizing green energy management, optimizing everything from grid stability to renewable energy forecasting. To stay ahead, it’s crucial to follow the innovations of leading players; a great resource for identifying them is this list of Top green energy companies to watch in 2025. Understanding their strategies will provide valuable insights into the future of AI’s role in a sustainable energy sector.
Improving Grid Stability and Resilience
The intermittent nature of renewable energy sources like solar and wind poses significant challenges to grid stability. AI offers solutions to mitigate these challenges by accurately forecasting renewable energy generation and adapting grid operations accordingly. Machine learning models can predict fluctuations in renewable energy output based on weather patterns and other relevant factors, allowing grid operators to preemptively adjust power generation and distribution to maintain a stable grid frequency and voltage.
This proactive approach minimizes the risk of blackouts and ensures continuous power supply, even during periods of high renewable energy variability. AI can also improve grid resilience by identifying and isolating faulty components quickly, reducing the impact of disruptions and facilitating faster restoration of power.
AI is revolutionizing green energy management, optimizing energy grids and predicting renewable energy generation. This is particularly relevant given the significant strides being made in Europe’s green energy sector, as detailed in this insightful report on Green energy initiatives in Europe. Ultimately, AI’s role in streamlining and enhancing the efficiency of these European initiatives will be crucial for achieving ambitious sustainability goals.
Challenges in Implementing AI-Based Optimization
Despite the numerous benefits, implementing AI-based optimization in existing power grids presents several challenges. The integration of AI requires substantial investment in advanced metering infrastructure (AMI), data analytics platforms, and skilled personnel. Data security and privacy are also crucial considerations, as AI algorithms require access to sensitive grid data. Furthermore, ensuring the reliability and explainability of AI algorithms is essential to build trust among stakeholders and regulatory bodies.
The complexity of power grid systems and the need for robust cybersecurity measures also pose significant hurdles.
AI Algorithms for Real-Time Grid Management and Fault Detection
Several AI algorithms are currently being used for real-time grid management and fault detection. Reinforcement learning (RL) algorithms are particularly well-suited for optimizing complex grid operations, allowing the AI system to learn optimal control strategies through trial and error. Support vector machines (SVMs) and neural networks are frequently employed for fault detection and prediction, analyzing sensor data to identify anomalies and predict potential failures.
These algorithms are often integrated into sophisticated grid management systems, enabling automated responses to grid events and improved decision-making by human operators.
Case Study: AI-Enhanced Grid Management in California
The California Independent System Operator (CAISO) has implemented AI-based tools to enhance grid management and integrate renewable energy more effectively. By using machine learning algorithms to forecast solar and wind power generation, CAISO has improved the accuracy of its energy forecasts, leading to better grid planning and resource allocation. This has resulted in reduced reliance on expensive peaking power plants and a decrease in the frequency and duration of grid imbalances.
The improved forecasting accuracy has also contributed to a reduction in the cost of energy procurement and enhanced grid stability. The implementation of AI-based tools at CAISO demonstrates the significant potential of AI to optimize grid operations and facilitate the transition to a cleaner, more sustainable energy system.
AI in Energy Efficiency and Conservation
Artificial intelligence (AI) is rapidly transforming the energy sector, offering significant potential for improving energy efficiency and conservation across various sectors. By leveraging machine learning algorithms and advanced data analytics, AI systems can analyze vast amounts of energy consumption data to identify patterns, predict future energy needs, and optimize energy usage in buildings and industrial facilities, ultimately leading to substantial cost savings and reduced environmental impact.AI analyzes energy consumption patterns through sophisticated algorithms that process data from various sources, including smart meters, building management systems (BMS), and industrial sensors.
These algorithms identify correlations between energy use and various factors like occupancy, weather conditions, equipment operation, and time of day. This detailed analysis reveals subtle trends and anomalies that might be missed by traditional methods, providing a granular understanding of energy consumption behavior. For instance, an AI system might detect unusually high energy consumption during off-peak hours, suggesting a potential equipment malfunction or inefficient process.
The ability to identify such anomalies allows for proactive intervention and targeted energy efficiency improvements.
AI Methods for Identifying Energy Savings
AI employs several methods to pinpoint areas for energy savings and optimization. Machine learning models, particularly supervised and unsupervised learning techniques, are commonly used. Supervised learning, for example, can be trained on historical energy consumption data to predict future energy needs and identify deviations from expected patterns. Unsupervised learning, on the other hand, can cluster similar energy consumption patterns to highlight areas with high energy intensity or inefficient operations.
Furthermore, anomaly detection algorithms identify unusual energy consumption spikes or dips, indicating potential equipment failures or operational inefficiencies that require attention. Reinforcement learning can optimize energy management strategies by learning through trial and error, adjusting control parameters in real-time to maximize energy savings while meeting operational requirements.
AI-Powered System for Automating Energy Savings in Smart Buildings
An AI-powered system for automating energy-saving measures in a smart building would integrate various sensors and actuators throughout the building to collect and analyze real-time data on energy consumption, occupancy, and environmental conditions. The system would utilize machine learning algorithms to predict energy demand based on historical data and external factors. This predictive model would then optimize the operation of building systems, such as HVAC (Heating, Ventilation, and Air Conditioning) and lighting, to minimize energy consumption while maintaining occupant comfort.
The system could dynamically adjust temperature setpoints based on occupancy levels, optimize lighting schedules according to natural light availability, and proactively identify and address equipment malfunctions that contribute to energy waste. The system’s performance would be continuously monitored and refined through feedback loops, ensuring optimal energy efficiency over time. For example, if a particular office space consistently shows high energy usage during unoccupied hours, the system could automatically adjust the HVAC and lighting settings to reduce consumption.
AI-Driven Solutions for HVAC and Lighting Control
AI significantly improves the efficiency of HVAC and lighting systems. In HVAC, AI algorithms can optimize temperature control based on occupancy patterns, weather forecasts, and building thermal characteristics, reducing energy consumption without compromising occupant comfort. For instance, AI can predict occupancy levels and preemptively adjust temperatures in unoccupied zones, saving considerable energy. In lighting control, AI can adjust lighting levels based on ambient light conditions and occupancy, ensuring adequate illumination while minimizing energy waste.
Smart lighting systems, combined with AI, can automatically dim or switch off lights in unoccupied areas, significantly reducing energy consumption. For example, a smart office building could use AI to automatically dim lights during daylight hours and adjust lighting intensity based on the number of people present in a room.
Environmental Benefits of AI-Driven Energy Efficiency
The implementation of AI-driven energy efficiency measures offers substantial environmental benefits. Before listing these benefits, it’s crucial to note that these benefits are realized through reduced energy consumption, which directly translates to lower greenhouse gas emissions.
- Reduced greenhouse gas emissions: Lower energy consumption directly translates to fewer greenhouse gas emissions from power generation, contributing to climate change mitigation.
- Decreased reliance on fossil fuels: Increased energy efficiency reduces the demand for fossil fuels, promoting the transition to cleaner and more sustainable energy sources.
- Improved air and water quality: Reduced emissions from power plants lead to improvements in air and water quality, benefiting both human health and the environment.
- Conservation of natural resources: Lower energy consumption reduces the need to extract and process natural resources used in energy production, promoting resource conservation.
AI for Sustainable Energy Resource Management
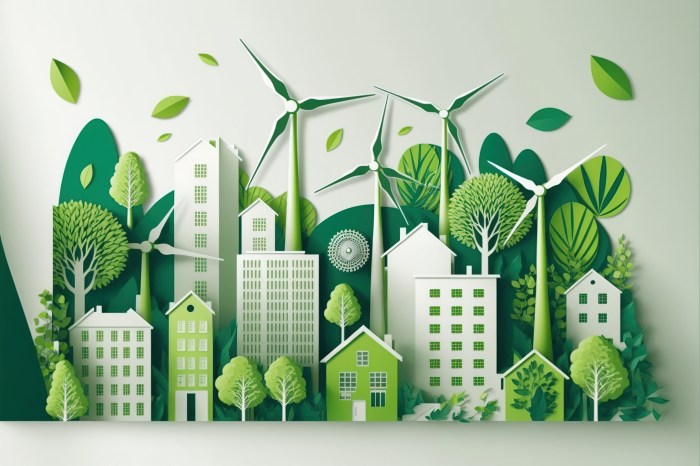
Source: neosnetworks.com
Artificial intelligence (AI) is rapidly transforming the sustainable energy sector, offering innovative solutions for optimizing resource management and enhancing the efficiency and reliability of renewable energy systems. The ability of AI to analyze vast datasets, identify patterns, and make predictions is proving invaluable in optimizing the operation and maintenance of renewable energy facilities, leading to significant cost savings and environmental benefits.AI’s role extends beyond simple data analysis; it enables proactive, predictive maintenance, minimizing downtime and maximizing energy production.
This proactive approach is crucial for the long-term sustainability and economic viability of renewable energy projects.
AI in Optimizing Renewable Energy Facility Operation and Maintenance
AI algorithms can analyze data from various sources – including sensor readings, weather forecasts, and historical performance data – to optimize the operation of renewable energy facilities. For instance, in solar farms, AI can adjust the tilt and orientation of solar panels based on real-time weather conditions, maximizing energy capture throughout the day. Similarly, in wind farms, AI can optimize the control of wind turbines to maximize energy output while minimizing wear and tear on the equipment.
This optimization extends to predictive maintenance, where AI algorithms can anticipate potential equipment failures and schedule maintenance before they occur, preventing costly downtime and ensuring consistent energy generation. Real-world examples include companies using AI to predict blade failures in wind turbines weeks in advance, allowing for timely repairs and preventing catastrophic failures.
AI-Driven Prediction and Prevention of Equipment Failures
Predictive maintenance using AI is revolutionizing the maintenance strategies for renewable energy assets. By analyzing historical data on equipment performance, alongside real-time sensor readings and environmental factors, AI algorithms can identify patterns indicative of impending failures. For example, in solar farms, AI can detect anomalies in the performance of individual panels, such as reduced power output or unusual temperature fluctuations, which could signal a developing fault.
In wind turbines, AI can detect vibrations or unusual sounds that indicate potential issues with the gearbox, blades, or generator. This early detection allows for proactive maintenance, preventing catastrophic failures and minimizing downtime. A specific example is the use of machine learning models to predict bearing failures in wind turbines with up to 90% accuracy, leading to significant reductions in maintenance costs and improved operational efficiency.
Challenges in Managing Geographically Dispersed Renewable Energy Assets
Managing geographically dispersed renewable energy assets presents significant challenges. Data collection from numerous remote locations can be complex and expensive, and ensuring data quality and consistency across different sites is crucial for accurate AI analysis. Communication infrastructure limitations in remote areas can also hinder real-time data transmission and analysis. Furthermore, integrating data from various sources, including different sensor types and formats, requires robust data integration and preprocessing techniques.
The diverse geographical conditions and weather patterns across different sites also necessitate the development of AI models that can adapt to varying environmental conditions. Successfully addressing these challenges requires a robust and scalable infrastructure for data acquisition, transmission, and processing, along with the development of AI algorithms that can handle noisy, incomplete, and heterogeneous data.
AI-Based Approaches for Optimizing Renewable Energy Grid Integration
Several AI-based approaches are employed to optimize the integration of renewable energy into the power grid. These include using machine learning algorithms to forecast renewable energy generation, enabling grid operators to better manage supply and demand. Another approach involves using AI to optimize the operation of energy storage systems, ensuring grid stability and reliability during periods of fluctuating renewable energy generation.
Furthermore, AI can be used to enhance grid management by optimizing power flow and minimizing transmission losses. Different AI techniques, such as deep learning, reinforcement learning, and expert systems, are employed depending on the specific application and data availability. For example, deep learning models are used for accurate forecasting of solar and wind power generation, while reinforcement learning algorithms are used to optimize the dispatch of renewable energy resources and the operation of smart grids.
Implementing an AI-Based Predictive Maintenance System for a Wind Farm
Implementing an AI-based predictive maintenance system for a wind farm involves a systematic approach.
- Data Acquisition and Preprocessing: Install sensors on wind turbines to collect data on various parameters such as vibration, temperature, wind speed, and power output. Clean and preprocess the collected data to handle missing values and outliers.
- Feature Engineering: Extract relevant features from the raw data that are indicative of potential equipment failures. This may involve using signal processing techniques to extract features from vibration data or applying statistical methods to identify anomalies in operational parameters.
- Model Development and Training: Develop and train a machine learning model using historical data and the engineered features. Various algorithms, such as support vector machines (SVM), random forests, or deep learning models, can be used depending on the data characteristics and the desired level of accuracy.
- Model Evaluation and Validation: Evaluate the performance of the trained model using appropriate metrics such as precision, recall, and F1-score. Validate the model on a separate dataset to ensure its generalization capability.
- Deployment and Monitoring: Deploy the trained model to a real-time monitoring system. Continuously monitor the model’s performance and retrain it periodically with new data to maintain its accuracy and effectiveness.
- Maintenance Scheduling and Optimization: Use the model’s predictions to schedule maintenance tasks proactively. Optimize the maintenance schedule to minimize downtime and maximize the utilization of the wind farm.
AI and the Development of New Green Energy Technologies
Artificial intelligence (AI) is rapidly transforming the landscape of green energy, accelerating the research, development, and deployment of sustainable energy technologies. Its ability to analyze vast datasets, identify patterns, and predict outcomes is proving invaluable in overcoming challenges that have historically hindered progress in this field. This section explores the multifaceted role of AI in fostering innovation within the green energy sector.
AI’s Acceleration of Sustainable Energy Technology Research and Development
AI algorithms are significantly shortening the research and development cycles for new sustainable energy technologies. By simulating various scenarios and predicting the performance of different materials and designs, researchers can efficiently screen numerous possibilities, identifying the most promising avenues for investigation. This drastically reduces the time and resources needed to bring innovative technologies to market. For example, AI is being used to optimize the design of new solar cell materials, leading to more efficient and cost-effective energy harvesting.
Similarly, AI is aiding in the discovery of new battery chemistries with improved energy density and lifespan, crucial for the wider adoption of electric vehicles and grid-scale energy storage.
AI in Materials Science for Enhanced Solar Cells and Batteries
AI is revolutionizing materials science, a crucial component in developing superior solar cells and batteries. Machine learning algorithms can analyze the vast chemical space of potential materials, predicting their properties and identifying those best suited for energy applications. This approach accelerates the discovery of novel materials with enhanced performance characteristics. For instance, AI is being used to design perovskite solar cells with higher efficiency and stability, surpassing the performance of traditional silicon-based cells.
In battery technology, AI helps optimize the composition and structure of electrode materials, leading to batteries with greater energy density, faster charging times, and longer lifecycles. This is exemplified by recent advancements in solid-state battery technology, where AI has played a key role in identifying promising electrolyte materials.
AI-Driven Innovations in Energy Storage and Transmission
AI is instrumental in improving the efficiency and reliability of energy storage and transmission systems. Smart grids, leveraging AI-powered algorithms, can optimize energy distribution, reducing transmission losses and improving grid stability. AI-driven predictive maintenance helps anticipate equipment failures in power plants and transmission lines, minimizing downtime and ensuring a continuous energy supply. Furthermore, AI is optimizing the operation of energy storage systems, maximizing their efficiency and lifespan.
Examples include AI-powered algorithms that manage the charging and discharging cycles of batteries in large-scale energy storage facilities, ensuring optimal energy storage and grid stabilization.
AI in the Design and Optimization of Novel Green Energy Systems
AI is playing a crucial role in the design and optimization of entirely new green energy systems. By simulating the performance of complex systems under various conditions, AI can identify optimal configurations and operating parameters. This is particularly relevant for integrated energy systems, combining renewable energy sources with energy storage and smart grid technologies. For example, AI can optimize the integration of solar, wind, and hydro power sources, maximizing energy output and minimizing reliance on fossil fuels.
AI is revolutionizing green energy management, optimizing processes from solar panel maintenance to grid stability. A key area for AI application is the efficient management of renewable energy projects, such as those detailed in this informative resource on Renewable energy projects in the US. Ultimately, AI’s role in predicting energy output and demand will be crucial for the continued success and expansion of green initiatives.
Moreover, AI can assist in the design of more efficient and cost-effective energy storage solutions, including pumped hydro storage, compressed air energy storage, and thermal energy storage.
AI in Next-Generation Wind Turbine Design
Imagine a next-generation wind turbine blade designed using AI. The process begins with AI algorithms analyzing vast datasets of wind patterns, weather conditions, and turbine performance data from existing turbines. This data is used to train a machine learning model that predicts optimal blade geometries for various wind speeds and directions. The AI then iteratively refines the blade design, simulating its performance under different conditions and optimizing for maximum energy capture and minimal structural stress.
The resulting blade is not only more efficient but also lighter and more durable, reducing manufacturing costs and maintenance requirements. The AI also optimizes the placement of the turbine within a wind farm, considering factors such as wind shear, turbulence, and the proximity of other turbines, maximizing the overall energy output of the farm. The control system of the turbine itself would also be AI-driven, dynamically adjusting the turbine’s operation in real-time to optimize energy capture based on instantaneous wind conditions.
This sophisticated approach leads to a significant improvement in energy generation and efficiency compared to traditional wind turbine designs.
Conclusion
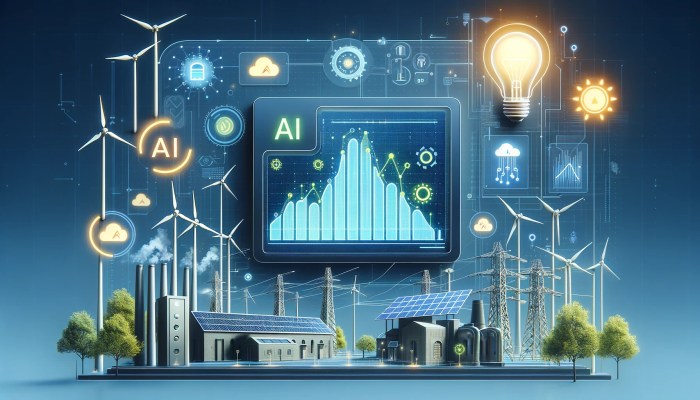
Source: framerusercontent.com
In conclusion, the application of AI in green energy management represents a significant leap forward in our pursuit of a sustainable energy future. Through advanced forecasting, optimized grid management, enhanced energy efficiency, and accelerated technological innovation, AI is proving to be a critical tool in tackling the challenges of climate change and ensuring a secure and reliable energy supply for generations to come.
The ongoing development and implementation of AI-driven solutions will undoubtedly shape the landscape of green energy, fostering a more efficient, resilient, and environmentally responsible energy sector.
FAQ Overview: AI In Green Energy Management
What are the ethical considerations of using AI in green energy?
Ethical considerations include data privacy, algorithmic bias impacting energy access, and the environmental impact of AI’s computational demands. Careful planning and responsible development are crucial.
How does AI contribute to reducing carbon emissions?
AI helps reduce carbon emissions by optimizing energy production and consumption, improving the efficiency of renewable energy sources, and predicting and preventing equipment failures, thus reducing waste.
What are the economic benefits of AI in green energy?
Economic benefits include cost savings from improved efficiency, reduced energy waste, increased renewable energy integration, and the creation of new jobs in the AI and green energy sectors.
What are the main barriers to wider adoption of AI in green energy?
Barriers include high initial investment costs, lack of skilled workforce, data scarcity or quality issues, and concerns about data security and privacy.